The passing out of scrubs on the first day of nursing orientation.
In finance, a uniform distribution is a distribution showing the probabilities of the outcomes of a random variable that has all equal (or roughly equal) probabilities for every single outcome. Since the probabilities of rolling any of the numbers 1 through 6 on a fair, six-sided die are all equal, the distribution of probabilities for the random variable of the outcome of rolling that six-sided die would be a uniform distribution...because each probability is equal to every other probability.
Just watch our videos on this one. Spielberg is so proud of having directed them.
Related or Semi-related Video
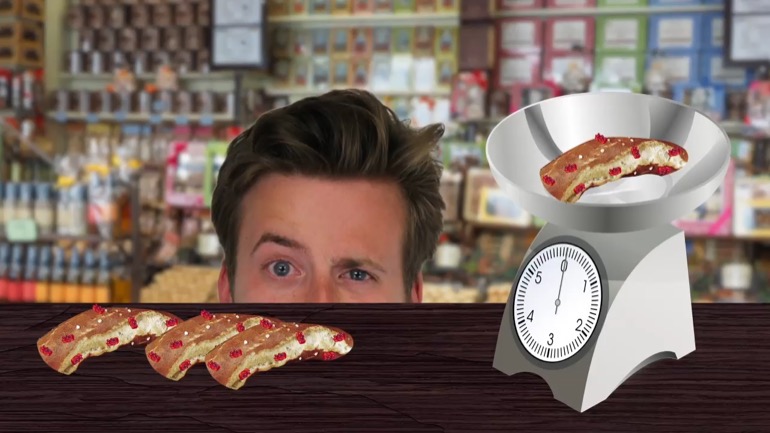
Finance: What is the standard normal dis...6 Views
And finance Allah shmoop What is the standard normal distribution
Senate Normal distribution is the destruction of the Z Scores
of the data points from a normal distribution Okay but
why do we need to create a new normal distribution
like the new normal Isn't that a thing Wasn't the
normal distribution we already had good enough before We explain
why the standard normal distribution is such a huge improvement
on the plain old normal distribution but we need a
quick recap of the original A normal distribution or normal
curve is a continuous bell shaped distribution that follows the
empirical rule which says that sixty eight percent of the
data is between negative one and one Standard deviations on
either side of the mean ninety five percent of the
data is between negative two and two Standard deviations on
either side of the mean and ninety nine point seven
percent of the data is between negative three and three
Standard deviations on either side of the mean well the
regular normal curve has its peak located at the mean
Ex Bar and is marked off in units of the
standard deviation s right there That's what it looks like
Adding the standard deviation over and over to the right
and subtracting the standard deviation over and over to the
left But what makes it normal The fact that sixty
eight percent of all the data is between one standard
deviation on each side of the means that makes it
normal It's that sixty eight percent truism that makes it
a normal distribution Then ninety five percent of the data
is between two standard deviations on either side of the
mean That's another test for normalcy And ninety nine point
seven percent of the data is between the three Senate
aviation's on either side Another test That's a third test
You passed all three your normal well tons of things
in nature and from manufacturing and lots of other scenarios
are normally distributed like heights of adult males or weights
of snicker bars or the diameter of drink cup lids
or eleventy million other things Okay fun size Snickers have
a mean weight of twenty point Oh five grams of
the standard deviation of point seven two grams and the
weights are normally distributed What that gives us this distribution
of fun size Snickers Wait it's the height of the
graph At any point it's the likelihood of us getting
a candy bar of that specific weight dire the curve
at a point the greater the chance we get the
exact weight This means that the fun size snickers wait
we'll get the most often is that twenty point Oh
five grams size that is smack dab in the middle
Right there waits larger and smaller than that will be
less common in our Halloween candy haul Waits like seventeen
point eight nine grams are twenty two point two one
grams will be extremely rare because there's shofar from the
middle and are at a part of the curve where
we have a very small likelihood of getting those weights
So why should we even mess with the normal distribution
we already have by calculating Z scores to create a
standard normal distribution And well what the heck is a
Z score Anyway We'll answer the first question in just
a sec but a Z scores of value we calculate
that tells us exactly how far a specific data point
is from the mean measured in units of standard deviation
Z scores were a way to get an idea for
how larger small a data point is compared to all
the other data points in the distribution It's like getting
a measure of how fast a Formula One racecar is
compared not to regular beaters on the road but two
other Formula One race cars the Formula One cars obviously
faster than the Shmoop mobile here But is it faster
than other Formula One cars That's what really matters A
Z score will tell us effectively where that one Formula
One car ranks compared to all the other ones we
can speed test If it's got a large positive Z
score it's faster than many if not most of the
cars It has a Z score close to zero Well
then it's right in the middle The pack speed wise
If it's got a small negative Z score well it's
the turtle to the other cars Hairs Why would we
plot the Z scores instead of the scores themselves Well
because the process of standardizing or calculating the plotting of
the Z scores of the data points makes any work
we need to do with the distribution about ten thousand
times easier When we calculated plot the Z scores we
create a distribution that doesn't care anything about the context
of the problem or about the individual means or standard
deviations or whatever Effectively we create one single distribution that
works equally well for heights of people or weights of
candy bars or diameters of drink lids or lengths of
ring tailed Leamer taels If we don't standardize by working
with Z scores we must create a normal curve that
has different numbers for each different scenario And we have
to do new calculations for each scenario for each different
set of values So let's explore the important features of
the standard normal distribution and how it differs from all
the other regular normal distributions The standard normal curve and
the regular normal curve look identical in shape They just
differ in how the X axis this thing right here
is divided Let's walk through an example where we compare
how the normal distribution of the actual data and the
standard normal distribution for the sea Scores of the data
are created at the same time Okay What are we
gonna pick here Well let's pick narwhal tusks They're very
close to normal in their distribution with a mean length
of two point seven five meters and standard deviation of
point to three meters The regular normal distribution of Narwhal
Tusk links are narwhal distribution is that I think we'll
have the peak located above the mean of two point
seven five meters We'll need the Z score of a
data point representing the length of two point seven five
to start labeling the standard normal distribution the same way
we'll Z scores were found by subtracting the mean from
a data point and dividing that value by the standard
deviation of the data To find a Z score we
subtract the mean two point seven five from our data
point also two point seven five to get zero And
then we divide that by the standard deviation of point
two three while we get a Z score for that
middle value of zero Here's the same normal curve of
the Tusk clanks paired with the standard normal curve of
the Z scores Now for the tick marks on the
straight up Tusk link distribution Right there we add the
standard deviation of point two three three times to the
mean of two point seven five to get the tick
marks to the right of the meanwhile we just get
was that two point nine eight and then three point
two ones were adding point to three to it And
then another point that gets us three point four four
There we go and we repeat that procedure on the
left but subtracted three times So we get to point
five to two point two nine And then what is
that two point Oh six on the left Well to
get these same values on our standard normal curve we
need to find some more Z scores The first score
of the right of the mean is that a value
two point nine eight meters It Z score will be
found by taking two point nine eight and subtracting the
mean of two point seven five to get that point
to three and then dividing that by the standard deviation
of point two three while we get one See that's
kind of a little mini proof there The second take
mark to the right will be for data points at
three point two one meters Well when we subtract the
mean we get point four six which we divide by
point two three and get Z equals two and the
third take mark their works out similarly gets a C
equals three See there it is Things will work out
similarly but negatively on the other side on the laughed
when we do the same thing for tick marks Negative
one negative too And then there we go Negative three
Well let's look at the two curves together One is
specific to the data of narwhal Tusk flanks while the
other is standardized to represent the perfect normal curve usable
for all normal data regardless of context or the values
of the means or standard deviations So after standardizing does
the standard normal curve follow the empirical rule Yeah it's
a normal curve After all it's even in the name
standard normal curve See they kind of tipped me off
to those things They're still sixty eight percent of data
points between Negative one and one on the standard normal
curve There's still ninety five percent of the data pretty
negative two and two on the standard normal curve And
there's still ninety nine point seven ten of the day
to pretty negative three and three on standard normal curve
so getting back to the ten thousand times easier thing
Well it comes in when we try to answer questions
like how many of the gummy coded pretzel logs weigh
between twelve and fifteen grams So here's the set up
Gummy coated pretzel log weights are normally distributed with a
mean of thirteen point two grams and a Sarah deviation
of point seven eight grams We want to know what
percentage of pretzel logs that come out of the gummy
bear coding machine way between twelve and fifteen grams which
the company considers their ideal weight range and likely that
customers wouldn't complain and send them back for being too
little or too big If we don't standardize things by
finding the Z scores of our boundary values of twelve
and fifteen grand we'll need some kind of technology to
interpret our mean standard deviation and boundary values in terms
of the normal curve specific to this situation If we
change anything about the problem like the boundary values or
mean or standard deviation well then we'll have to re
input all the new data and start completely over And
that would suck On the other hand since we know
that data are already normally distributed While we can simply
standardize the two boundary values by calculating their Z scores
and use the majesty of the Z table this thing
to answer our questions which is a table telling us
what percentage of data lies to the left or right
of an easy score across the whole standard normal distribution
Many lives were lost and billions of dollars were spent
Teo build this thing so you know you gotta respect
it not to put too fine a point on it
but if we don't standardize dizzy scores we need to
use a unique normal curve and unique calculations every single
time we work with those situations But if we do
standardized to Z scores we just need to check the
one table for every situation It's like choosing to go
to a different store every time we need a different
product or going toe one store that has all of
them in one place like you'd rather go to Safeway
than just the broccoli store and then the egg store
and then the milk store right So let's calculate our
two Z scores for our boundary values and then check
the Z Table to get our percentage of pretzel logs
in the sweet spot that twelve to fifteen range thing
What will take first data point twelve and subtract the
mean weight of thirteen point to giving us negative one
point two grams and then divide that by the standard
deviation of point seven eight which gives us a Z
score there of negative one point five three eight Then
we'll take the second data point fifteen subtract that mean
of thirteen point two to get one point eight then
divide that value by our standard deviation of point seven
eight to get his E score of two point three
eight Well there are two different kinds of ze tables
One shows the area to the left of a specific
Z score The other shows the area to the right
They both give the same info just so we'll use
a left ze table A Siri's of Z scores accurate
to the tense place runs down the left hand side
and the hundreds place for each of those e scores
runs across the top Well the percentage of data to
the left of a specific Z score can be found
at the intersection of a row and a column bullied
around both our Z scores to the hundreds Place negative
one point five four and then two point three one
respectively in order to locate a percentage of data to
the left of each one Well we'll go down to
the negative one point five row then across to the
column here headed by the negative zero point zero four
where negative one point five Avenue intersects with negative zero
point zero four street and we find a percentage of
data to the left of Z equals negative one point
five four of zero point zero six one seven eight
This thing Well well then head way down to the
two point three boulevard then across to the point zero
one road they cross at point nine eight nine five
six So now what What do we do with these
Two percentage is well glad you asked We know the
percentage of data to the left of our fifteen grand
upper boundary Which is that a Z score of two
point three one We also know the area to the
left of our twelve Graham lower boundary at a Z
score of negative one point five four announced time to
merge those two areas Check the area to the left
of the Z score of two point three one on
the standard normal curve This is the percentage of data
to the left of that value Now check the area
to the left of it Z score of negative one
point five four on the same standard normal curve Well
this is the percentage of data to the left of
that value If we cut away the area to the
left of Z equals negative one point five four or
left with the area here between Z equals negative one
point five for ends e equals two point three one
This is the percentage of data between these two values
and you're looking at this really heavily to be sure
that you got enough in that general sweet spot range
They don't get a whole lot of returns from angry
customers Well we just need to subtract the point Oh
six one seven eight from the point nine eight nine
five six to get the percentage of data between those
two values which is yes about ninety three percent so
What does that mean Well that means ninety three percent
of the gum encoded pretzel logs produced will be between
twelve and fifteen grams in weight And that's either good
news or not Well a couple of important safety tips
though Before you all head out to the store for
some more gumming coded pretzel log We should on Lee
try to standardize I'ii do things with Z scores if
the data are normal in shape to begin with If
they're not the data Maki nations here will be useless
to you Make sure you're paying attention to what kind
of ze table you have again Some show areas to
the left while others give areas to the right and
specific Z scores Every time you've got a set of
normally distributed data you should standardize the situation by finding
Z scores And while you'll save yourself a ton of
work in the long run what least tons of stats
work if we can't help you Sorry I do
Up Next
What is the normal distribution/normal curve? The normal distribution or normal curve is when data transposed into a graph shows a fairly strong ad...